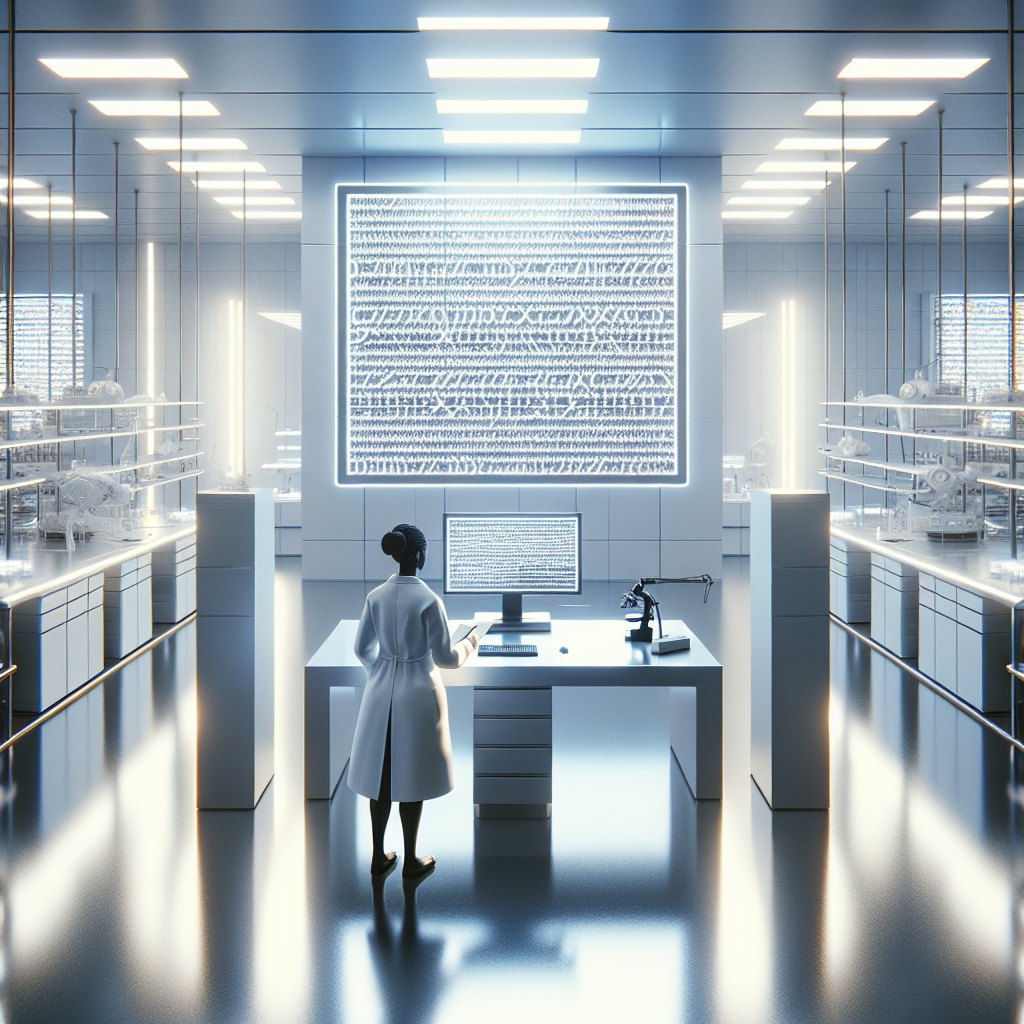
This AI Paper from the University of Washington, CMU, and Allen Institute for AI Unveils FAVA: The Next Leap in Detecting and Editing Hallucinations in Language Models
Main Ideas:
1. Large Language Models (LLMs) have gained popularity for their human-imitating skills.
– LLMs are advanced AI models that can answer questions, complete code, and summarize text, among other tasks.
– They leverage the power of Natural Language Processing (NLP) and Natural Language Generation (NLG).
2. FAVA is a new system developed by researchers from the University of Washington, CMU, and Allen Institute for AI.
– FAVA (False Assertion Visualizer and Analyzer) is designed to detect and edit hallucinations in LLMs.
– Hallucinations refer to instances where LLMs generate false or unreliable information.
– FAVA allows users to visualize and analyze false assertions to identify and correct inaccuracies.
3. FAVA’s architecture is based on a two-stage process.
– The first stage involves detecting false assertions in LLM outputs.
– The second stage focuses on editing the hallucinations through a reinforcement learning-based approach.
4. FAVA demonstrates promising results in detecting and editing hallucinations in LLMs.
– FAVA outperforms existing methods in identifying false assertions.
– It successfully reduces hallucination rates and improves model output quality.
Author’s Take:
Researchers from the University of Washington, CMU, and Allen Institute for AI have developed FAVA, an innovative system that aims to detect and edit hallucinations in Large Language Models (LLMs). LLMs have gained popularity for their human-like abilities, but they can sometimes generate false or unreliable information. FAVA addresses this issue by providing a two-stage process for detecting and editing hallucinations. With promising results in reducing hallucinations and improving model output quality, FAVA represents a significant step forward in enhancing the trustworthiness of LLMs.
Click here for the original article.