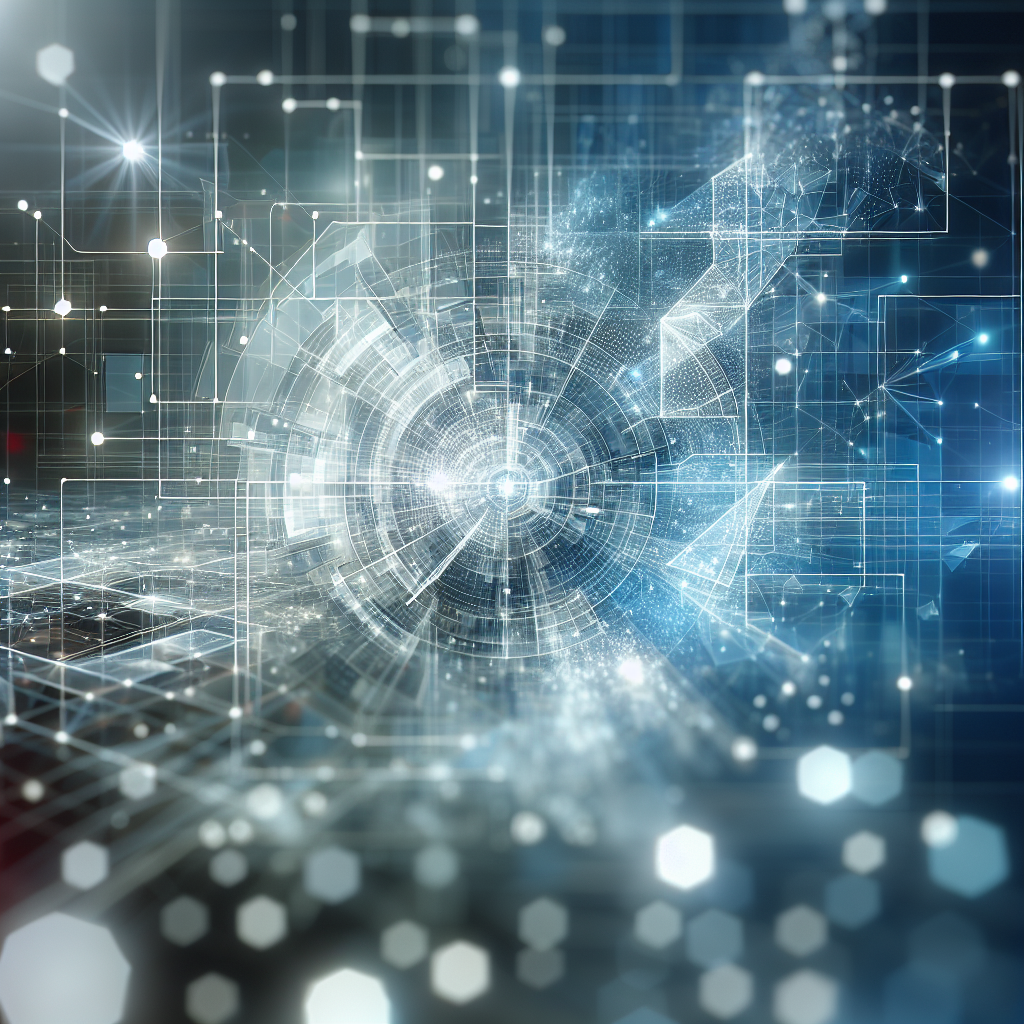
Summary of “KAIST and DeepAuto AI Researchers Propose InfiniteHiP Framework”
Main Points:
– Large language models (LLMs) face challenges with processing extended input sequences like significant computational and memory resources, slow inference, and high hardware costs.
– The attention mechanism in LLMs exacerbates these challenges due to its quadratic complexity compared to sequence length.
– Researchers from KAIST and DeepAuto AI have introduced InfiniteHiP, a long-context LLM framework designed for 3M-token inference on a single GPU.
– InfiniteHiP reduces the overhead of processing extended context by utilizing a new position-sensitive attention mechanism.
Author’s Take:
The introduction of InfiniteHiP by KAIST and DeepAuto AI marks a significant step towards addressing the challenges faced by large language models. By focusing on optimizing long-context processing and reducing computational overhead, this innovative framework may pave the way for more efficient and cost-effective LLM implementations in the future.
Click here for the original article.