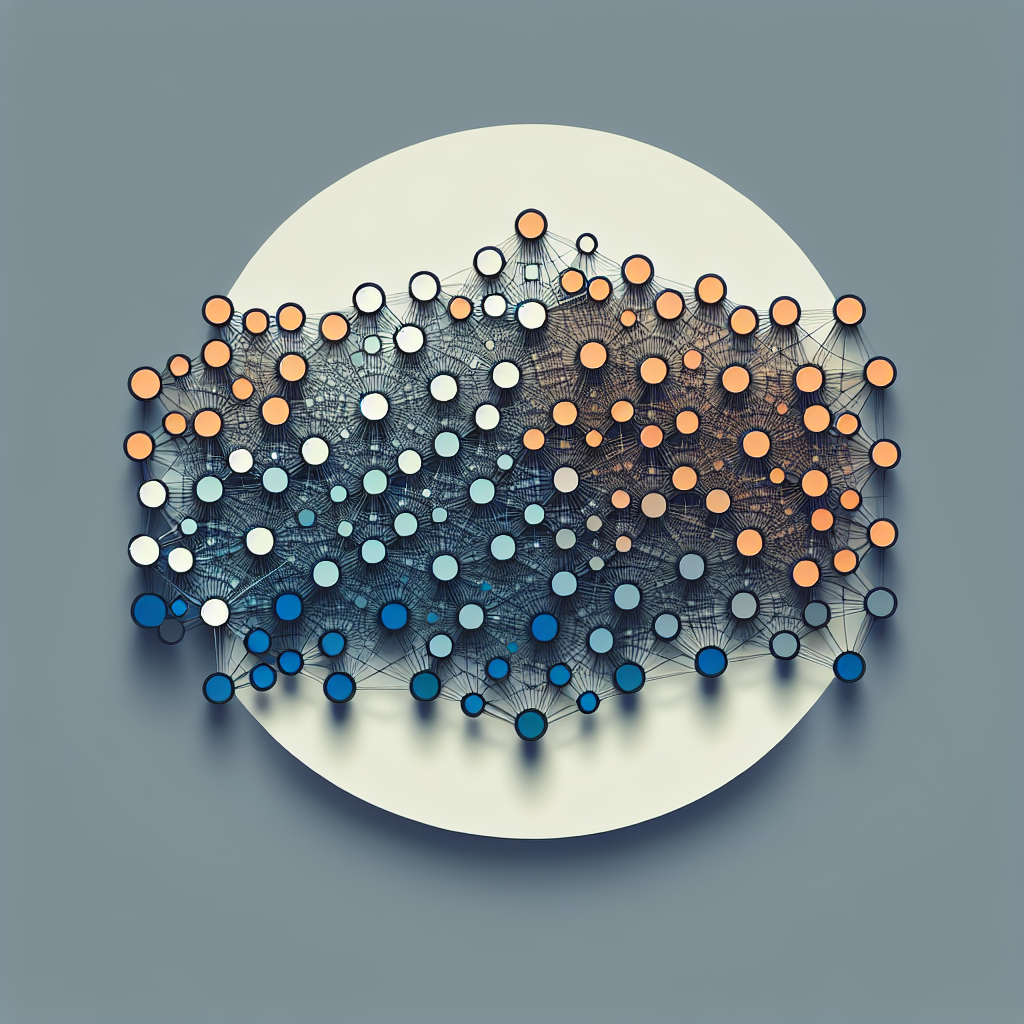
# Summary of the article “Higher-Order Guided Diffusion for Graph Generation: A Coarse-to-Fine Approach to Preserving Topological Structures”
## Main Ideas:
– Graph generation involves creating structured, non-Euclidean representations with meaningful relationships between entities.
– Existing methods often struggle to capture higher-order interactions crucial for applications like molecular modeling and social network analysis.
– Diffusion-based techniques, initially used in image synthesis, have gained popularity for generating graphs.
## Author’s Take:
In the realm of graph generation, the introduction of diffusion-based methods offers a promising solution to the challenges of capturing intricate higher-order interactions necessary for diverse applications. This innovative approach signifies a significant advancement towards retaining topological structures in graph generation tasks.
Click here for the original article.