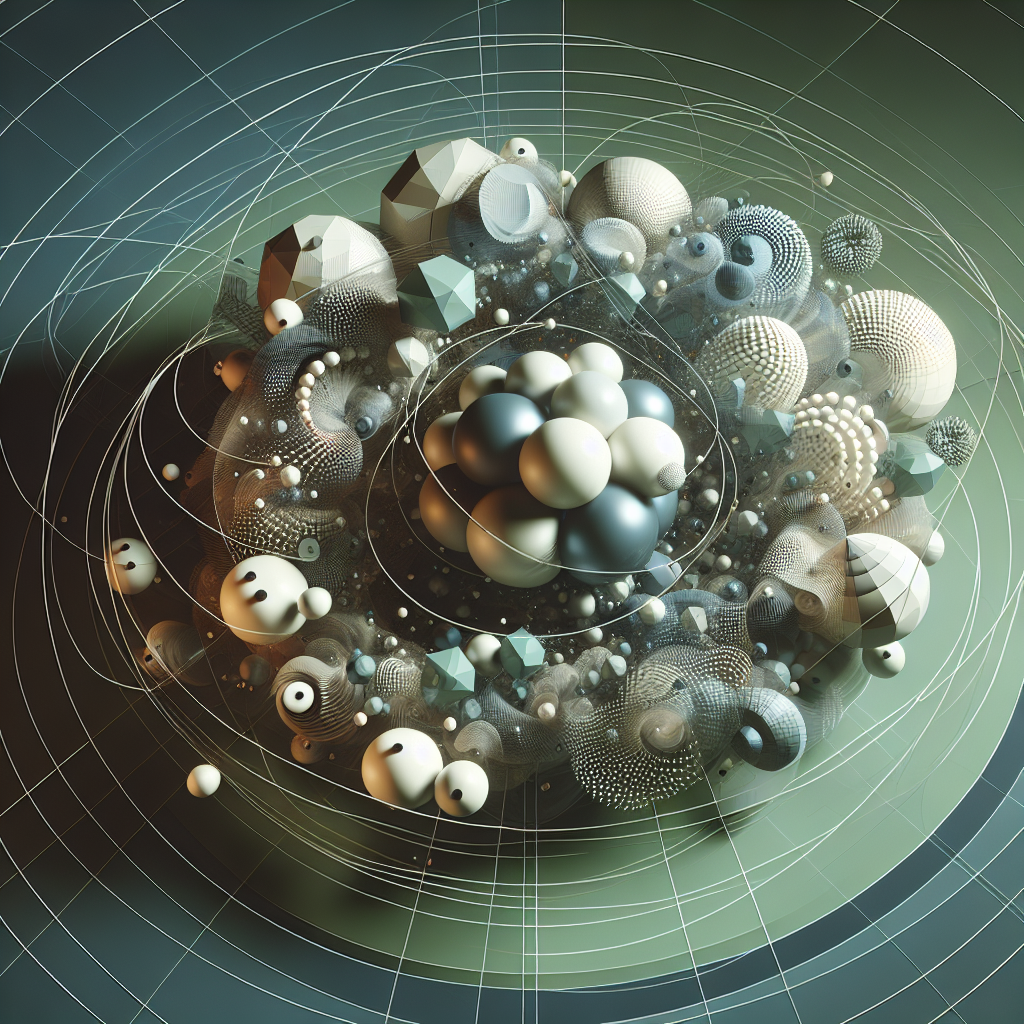
Summary:
– Machine learning is advancing into non-Euclidean spaces to capture complex geometric properties of data.
– Non-Euclidean representation learning involves embedding data into hyperbolic, spherical, or mixed-curvature product spaces.
– These approaches are beneficial for modeling structured and hierarchical data.
Author’s Take:
Machine learning is breaking boundaries with non-Euclidean representation learning, showcasing its versatility in capturing intricate geometric patterns. Columbia University’s AI paper introduces Manify, a Python library that empowers researchers to delve deeper into non-traditional data structures, opening doors to new possibilities in the field of artificial intelligence.
Click here for the original article.