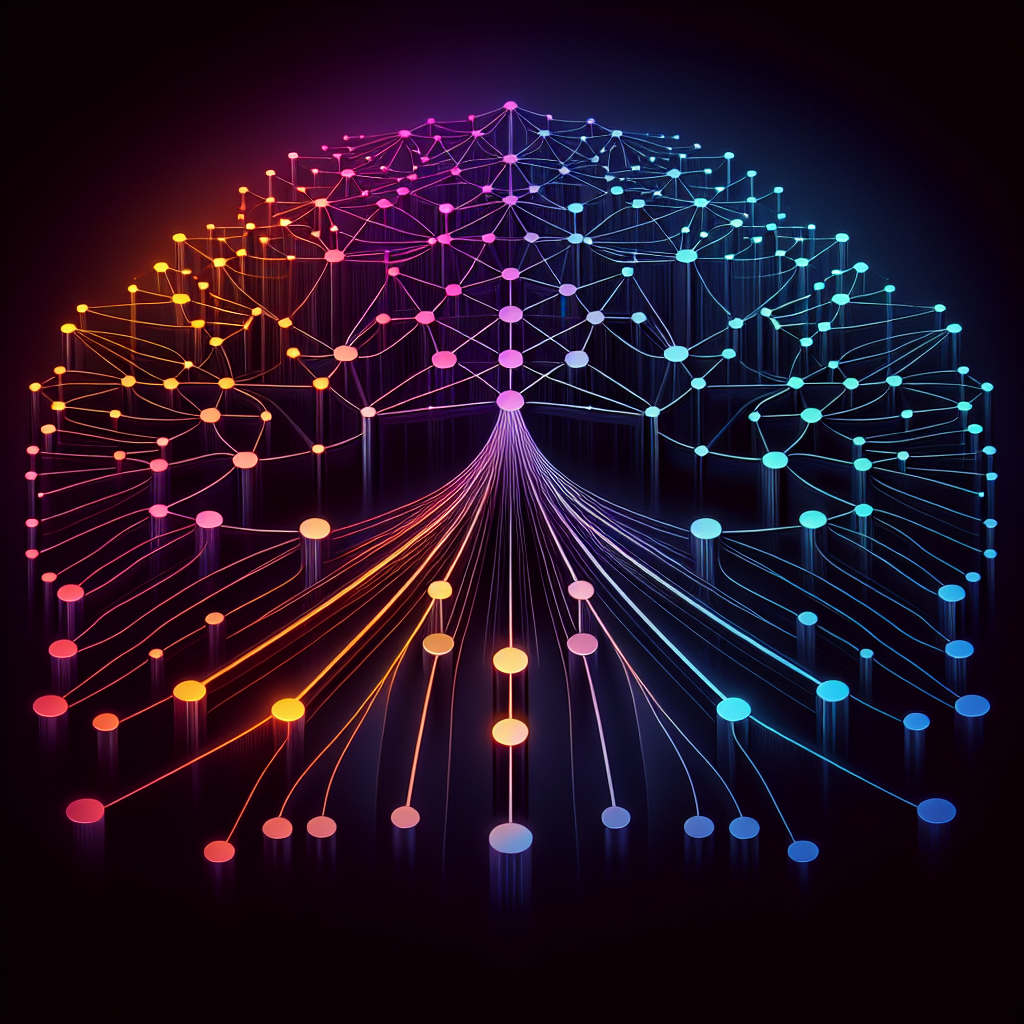
Summary:
– Recent advancements in Large Language Models (LLMs) have improved reasoning capabilities through Reinforcement Learning (RL) fine-tuning.
– LLMs undergo RL post-training after initial supervised learning for token prediction to improve reasoning outcomes.
– The RL post-training process allows LLMs to explore multiple reasoning paths akin to how agents navigate a game, leading to emergent behaviors like self-correction.
Author’s take:
The integration of Reinforcement Learning post-training with Large Language Models represents a significant leap in enhancing reasoning capabilities, showing promise for more concise and accurate outcomes in AI-powered models. This approach not only boosts the efficiency of language models but also opens doors for further advancements in natural language processing tasks.
Click here for the original article.