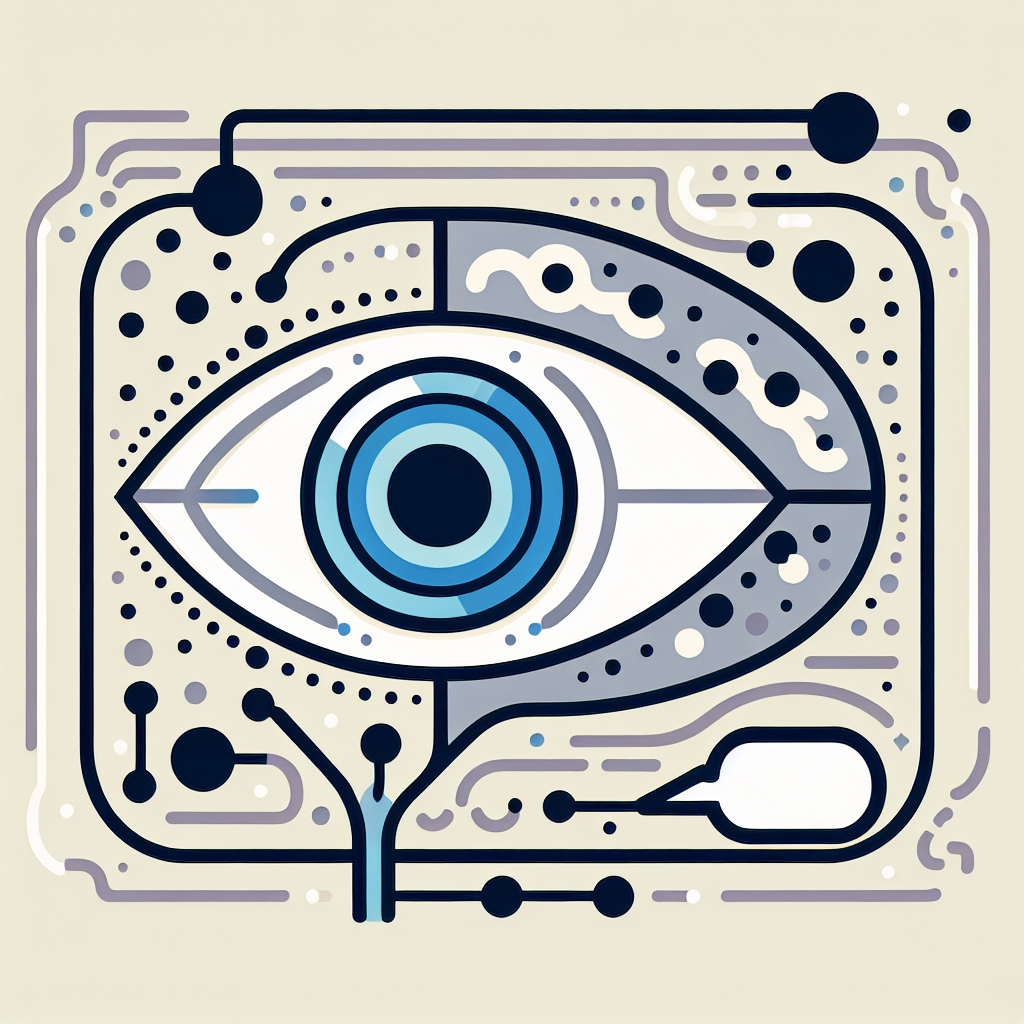
Enhancing Vision-Language Models with Chain of Manipulations: A Leap Towards Faithful Visual Reasoning and Error Traceability
Main Ideas:
- Big Vision Language Models (VLMs) have shown effectiveness in visual question answering, visual grounding, and optical character recognition.
- Humans mark or process the provided photos to improve convenience and accuracy.
- Researchers propose enhancing VLMs with a chain of manipulations to enable faithful visual reasoning and error traceability.
- This approach allows for better understanding of the model’s decision-making process and identification of potential errors.
- The proposed framework includes three key components: image manipulation operators, a detector network, and an error traceability module.
Author’s Take:
The integration of a chain of manipulations into Vision-Language Models has the potential to greatly improve visual reasoning and error traceability. By allowing for a better understanding of the decision-making process of these models, it becomes easier to assess their accuracy and identify potential errors. This framework could be a significant step forward in enhancing the capabilities of Vision-Language Models and ensuring their reliability.