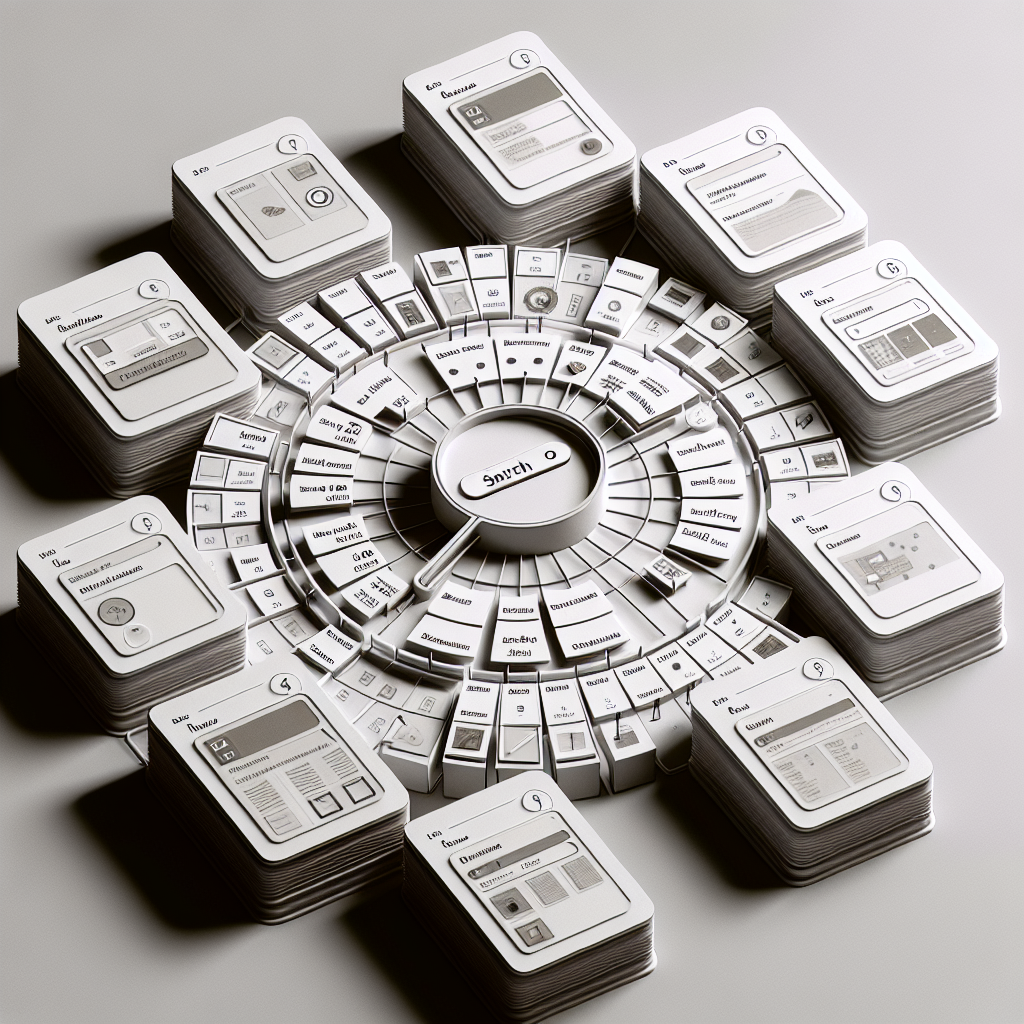
Researchers from San Jose State University Propose TempRALM: A Temporally-Aware Retriever Augmented Language Model (Ralm) with Few-shot Learning Extensions
Main Ideas:
- Researchers from San Jose State University have proposed TempRALM, a temporally-aware retriever augmented language model (Ralm) with few-shot learning extensions.
- TempRALM aims to enhance the retrieval and understanding of information from the web by factoring in temporal aspects.
- This approach allows for the identification and retrieval of specific information from different historical periods.
- The researchers propose using a combination of pretrained language models and a method called “query value decomposition” to improve the few-shot learning capabilities of TempRALM.
- Initial experiments with TempRALM have shown promising results in retrieving temporally relevant information.
Author’s Take:
TempRALM, a proposed language model from San Jose State University, aims to improve the retrieval and understanding of information from the web by considering temporal aspects. By factoring in the historical context, TempRALM enables the identification and retrieval of specific information from different time periods. The addition of few-shot learning extensions further enhances its capabilities. This research could have significant implications for information retrieval and knowledge exploration on the web.