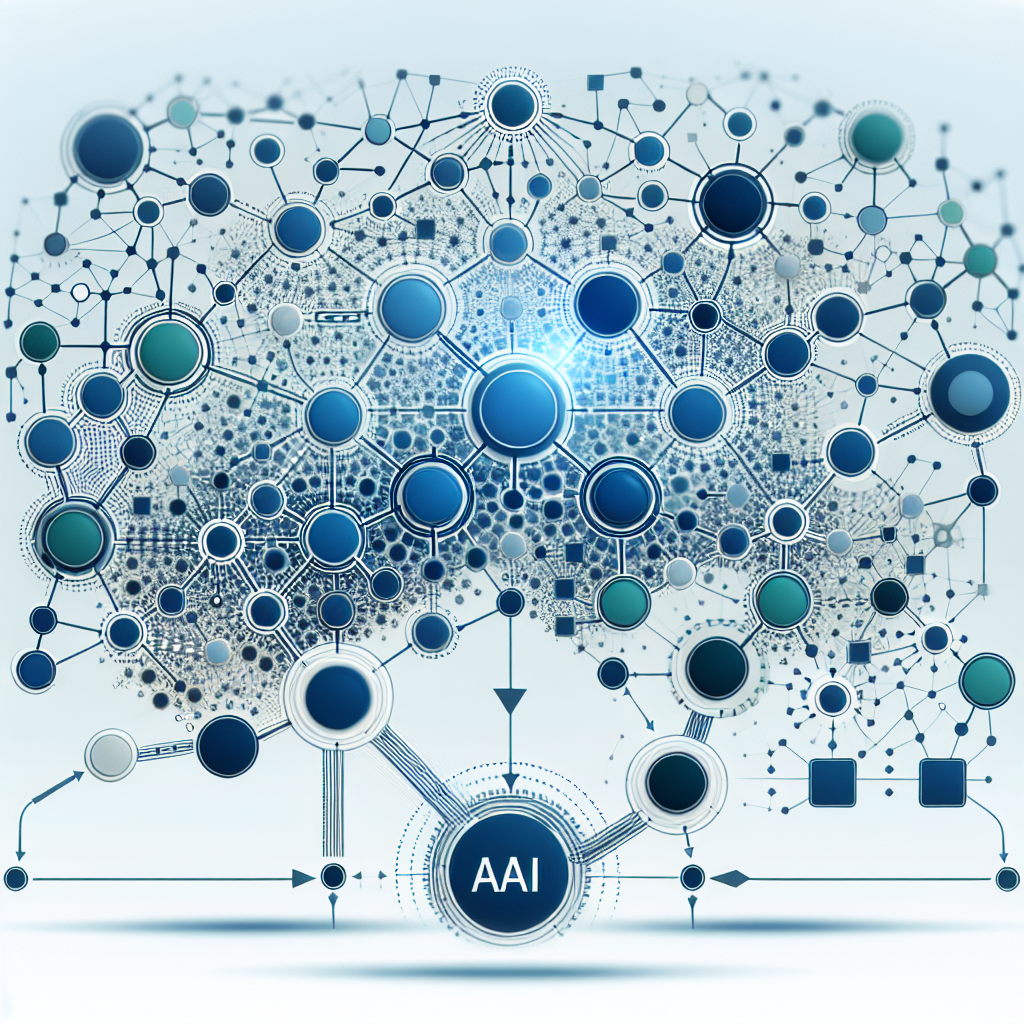
# Summary of the Article:
– **Focus**: Large language models (LLMs) emphasize refining chain-of-thought (CoT) reasoning to generate coherent outputs.
– **Challenge**: Generating structured reasoning responses in LLMs often demands significant computational resources and large datasets.
– **Solution**: A new data-efficient approach introduced by UC Berkeley aims to enhance long chain-of-thought reasoning in LLMs.
## Author’s Take:
UC Berkeley’s innovative data-efficient approach marks a significant step towards streamlining long chain-of-thought reasoning in Large Language Models, addressing the challenge of resource-intensive structured reasoning generation.
Click here for the original article.