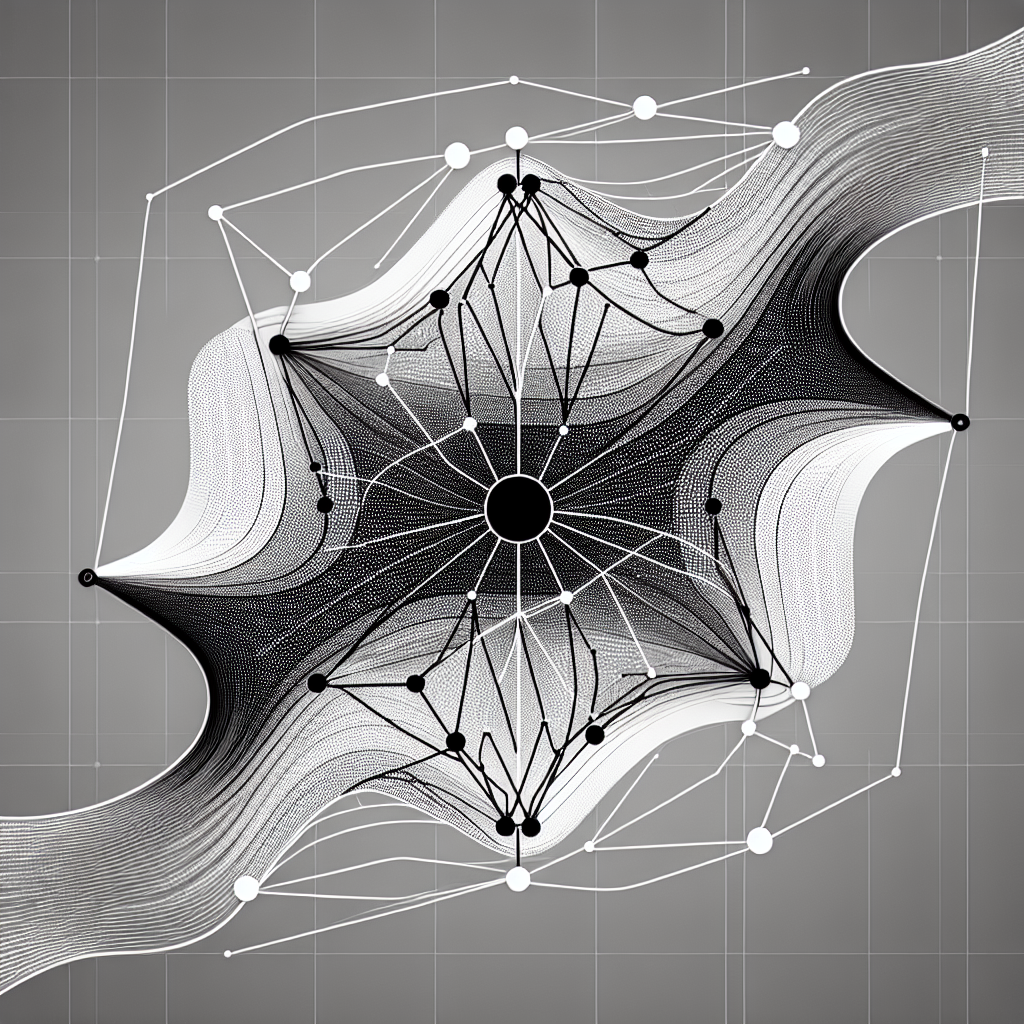
This AI Paper Proposes COPlanner: A Machine Learning-based Plug-and-Play Framework that can be Applied to any Dyna-Style Model-based Methods
Summary:
- Model-based reinforcement learning (MBRL) faces challenges in managing imperfect dynamics models, leading to suboptimal policy learning in complex environments.
- Researchers propose COPlanner, a plug-and-play framework that uses machine learning to improve the accuracy of model predictions and ensure adaptability.
- COPlanner utilizes the Dyna-style model-based methods and combines them with learned transition models, leading to better policy learning.
- This framework is validated on various benchmark tasks, demonstrating its efficacy in improving model accuracy and policy learning.
Author’s Take:
This AI paper introduces COPlanner, a machine learning-based framework that addresses the challenge of managing imperfect dynamics models in model-based reinforcement learning. By leveraging learned transition models and Dyna-style methods, COPlanner improves the accuracy of model predictions. This plug-and-play framework demonstrates promising results in benchmark tasks, offering a potential solution for enhancing policy learning in complex environments.