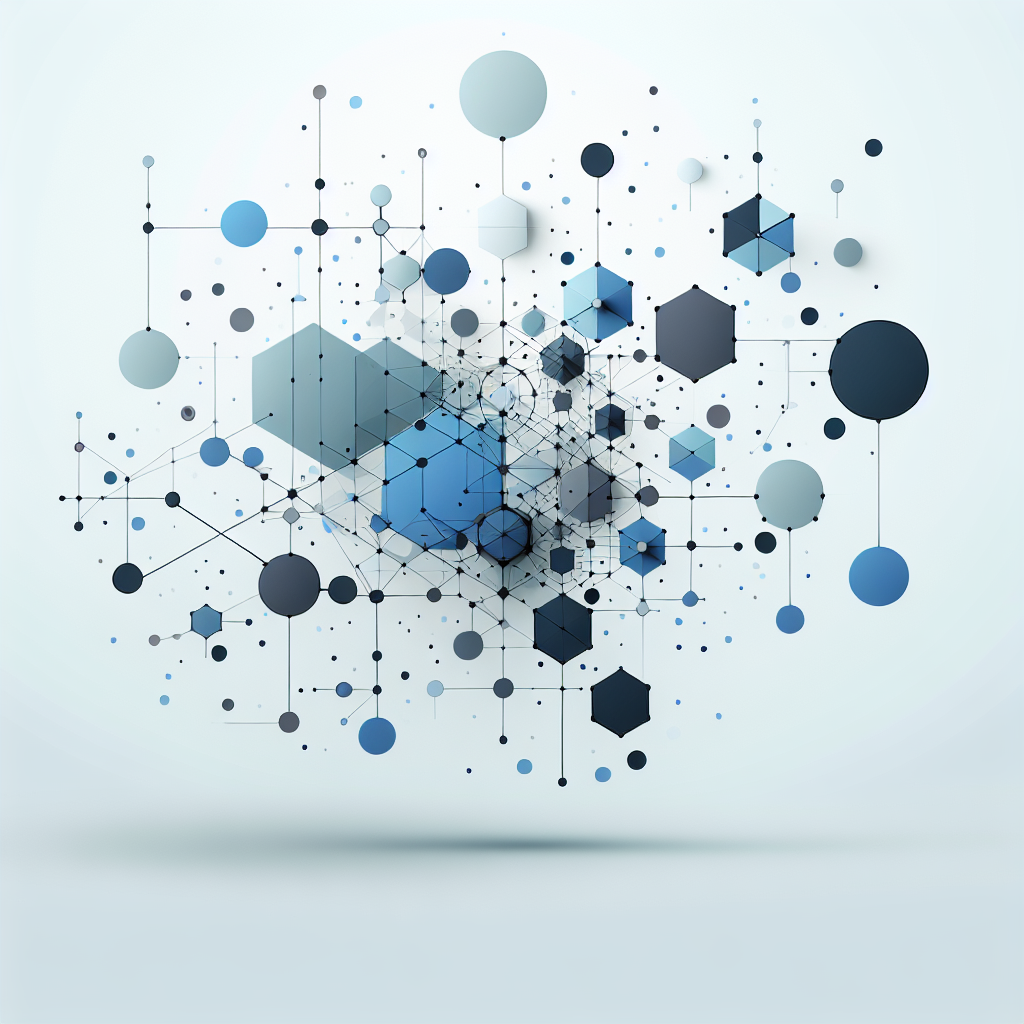
Main Ideas:
– Lexicon-based embeddings are considered a good alternative to dense embeddings.
– However, these embeddings face challenges that hinder their broader use, such as tokenization redundancy.
– One notable issue is that subword tokenization can separate tokens that are semantically equivalent, leading to inefficiencies and inconsistencies in the embeddings.
– Another limitation mentioned is the unidirectional attention in causal language models, restricting tokens from fully utilizing…
Author’s Take:
Lexicon-based embeddings offer a promising alternative to dense embeddings, but obstacles like tokenization redundancy and unidirectional attention in language models impede their widespread adoption. Efforts to address these challenges could significantly enhance the effectiveness and applicability of lexicon-based text embeddings in various AI applications.
Click here for the original article.