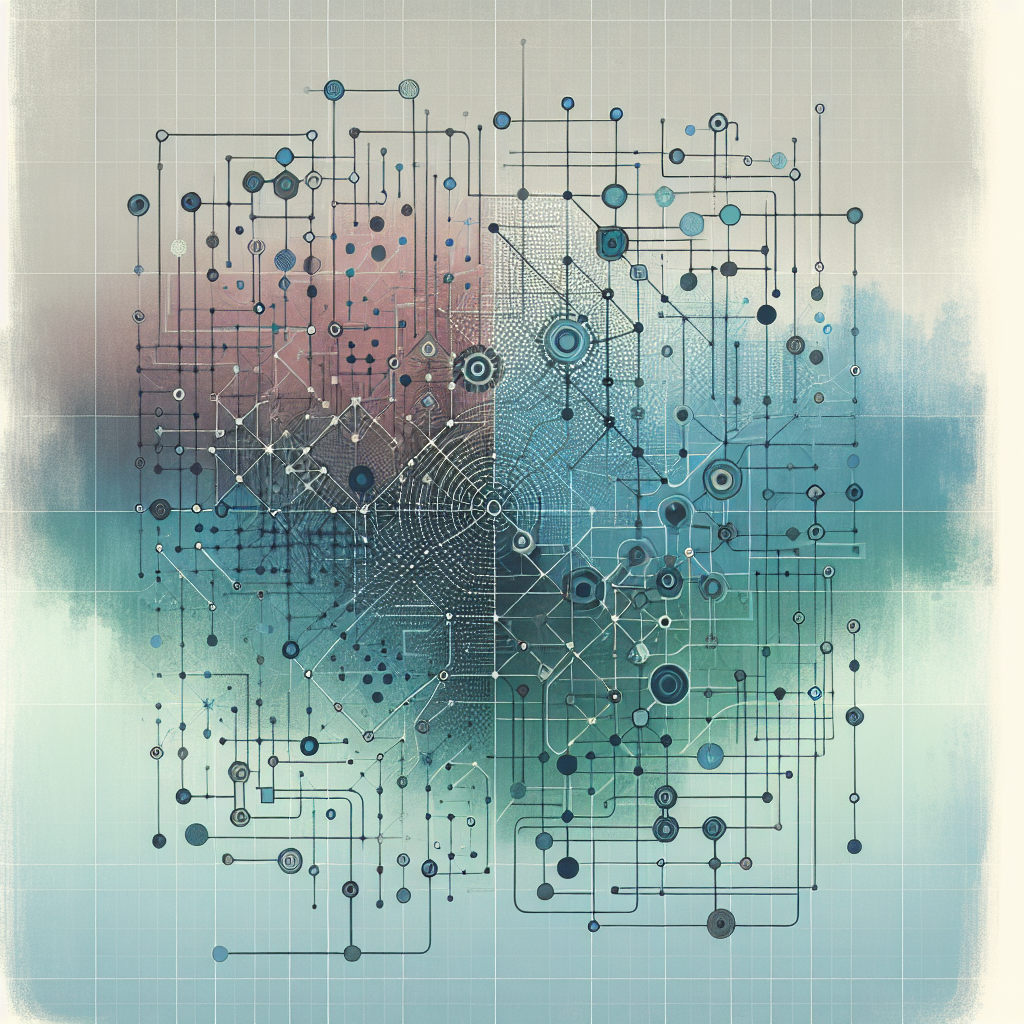
This AI Paper from UCLA Revolutionizes Uncertainty Quantification in Deep Neural Networks Using Cycle Consistency
Main Ideas:
- Deep neural networks are widely used in various fields, including data mining and natural language processing.
- Deep learning is also used in solving inverse imaging problems, such as image denoising and super-resolution imaging.
- However, deep neural networks often suffer from inaccuracies.
- Researchers from UCLA have developed a new approach called Cycle Consistency to improve uncertainty quantification in deep neural networks.
Summary:
Researchers from UCLA have published a paper describing a new approach called Cycle Consistency that aims to improve uncertainty quantification in deep neural networks. Deep learning is extensively used in various fields, but it often suffers from inaccuracies. The UCLA researchers’ approach uses cycle consistency to enhance uncertainty estimation in deep neural networks. This new technique could have significant implications for improving the accuracy and reliability of deep learning models in tasks such as image denoising and super-resolution imaging.
Author’s Take:
Deep neural networks have become a powerhouse in various fields, but their inaccuracies have been a major challenge. The cycle consistency approach developed by UCLA researchers could revolutionize uncertainty quantification in deep neural networks, addressing this challenge. By improving uncertainty estimation, deep learning models could become more reliable and accurate, enhancing their performance in tasks such as image denoising and super-resolution imaging. This research paves the way for advancements in using deep learning techniques in solving inverse imaging problems.
Click here for the original article.